Unleashing the Potential of Large Language Models through Reading Comprehension: Insights from Oxford AI Solutions
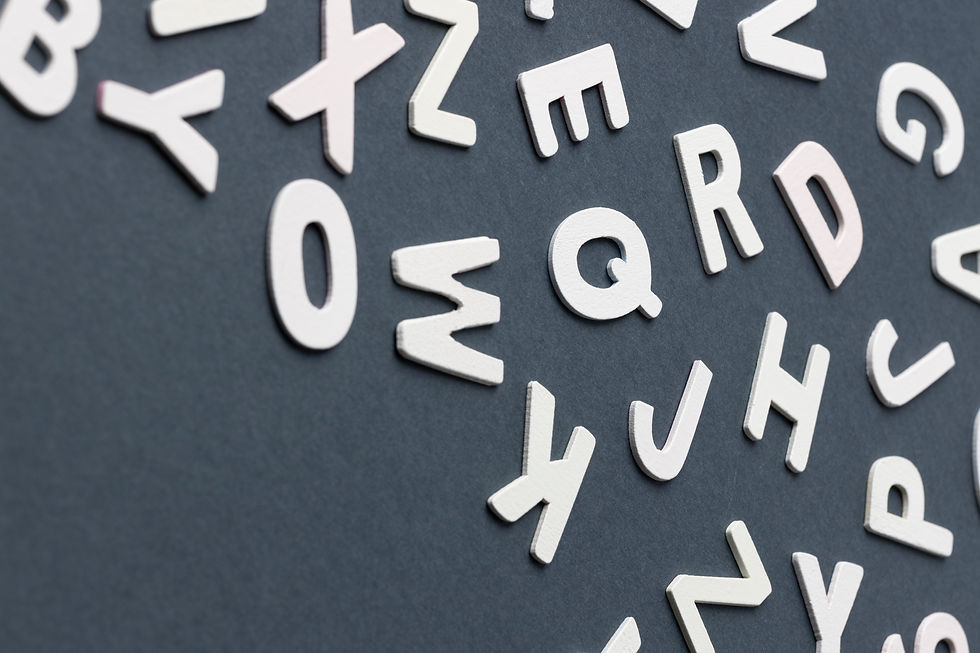
6/6/24, 9:00 PM
In the rapidly evolving landscape of artificial intelligence, Oxford AI Solutions is at the forefront of pioneering advancements that leverage innovative methods to adapt large language models (LLMs). Our latest research explores how integrating reading comprehension techniques into the training of LLMs can significantly enhance their performance, especially in domain-specific tasks. This article delves into our revolutionary approach, its implications, and the transformative potential it holds for various industries.
The Challenge: Domain-Specific Pre-Training
Traditional pre-training on domain-specific corpora endows LLMs with substantial domain knowledge but often at the expense of their general prompting abilities. This paradox presents a significant challenge: how can we retain the specialized knowledge without compromising the model's ability to generalize across diverse tasks?
Understanding Domain Knowledge in AI: Pre-training LLMs on specialized datasets, such as medical journals for biomedicine or financial reports for finance, enhances their capability to understand and process domain-specific information. However, this focused training can lead to a narrow understanding that reduces the model's flexibility and adaptability to other contexts.
The Need for Balance:Achieving a balance between domain-specific expertise and generalizability is crucial for creating versatile AI systems. This balance ensures that the model can apply its knowledge across various scenarios without losing the ability to handle specific, nuanced tasks effectively.
Our Solution: Transforming Raw Corpora into Reading
Comprehension Tasks
Inspired by human learning, where reading comprehension exercises enhance knowledge retention and application, we developed a method to convert raw corpora into structured reading comprehension tasks. This method involves enriching each text with a series of tasks that are directly related to its content. These tasks include summarization, word-to-text generation, natural language inference, commonsense reasoning, and text completion.
Detailed Process:
1. Summarization:
Task: Generate a concise summary of the provided article.
Example: For a medical journal article on a new treatment, the model summarizes the key findings and implications.
2. Word-to-Text Generation:
Task: Generate sentences incorporating specific domain-related keywords.
Example: Using keywords like "gene therapy," "clinical trial," and "outcome," the model constructs informative sentences.
3. Natural Language Inference:
Task: Determine the relationship between pairs of sentences (entailment, contradiction, or neutrality).
Example: Assess whether a statement about a financial trend logically follows from a previous analysis.
4. Commonsense Reasoning:
Task: Understand and predict cause-and-effect relationships within the text.
Example: Predict the consequences of a new regulatory policy on market behavior.
5. Text Completion:
Task: Complete a text based on its beginning or middle.
Example: Given an introduction to a legal case, the model predicts potential outcomes based on precedent and context.
Regex-Based Pattern Identification: We use regex-based patterns to identify key sentences within a text, generating diverse tasks from these sentences. Each task prompts the model to apply its understanding in various ways, thereby reinforcing its knowledge and improving its ability to handle related queries effectively.
Key Innovations and Methodology
1. Scalability and Versatility:
Our method is highly scalable and can be applied to any pre-training corpora. This flexibility allows it to be used across different domains, from biomedicine to finance and law.
Scalability in Action: By applying this method to various domains, we ensure that the LLMs developed are not only experts in their specific fields but also capable of generalizing their knowledge.
2. Enhanced Performance:
By converting texts into reading comprehension tasks, we consistently enhance the model's performance. Our 7B language model, for instance, achieves competitive performance with much larger domain-specific models such as BloombergGPT-50B.
Performance Metrics: We use comprehensive benchmarks to evaluate the model's capabilities, ensuring that it meets high standards across all tasks.
3. Generalization Across Domains:
The improvements are not limited to domain-specific tasks. The reading comprehension-based training also boosts the model's performance on general benchmarks, demonstrating its broad applicability and potential for creating versatile AI systems.
Broad Applicability: The ability to perform well on general benchmarks indicates the model's potential for widespread application across various industries.
Practical Applications and Results
In our experiments, we evaluated the efficacy of our approach across three primary domains: biomedicine, finance, and law. The results were compelling:
Biomedicine:
Tasks: PubMedQA, ChemProt
Performance: The model excelled in handling complex biomedical queries with high accuracy, demonstrating its capability to process and understand intricate medical information.
Example Application: Assisting researchers in quickly summarizing new studies and identifying potential areas for further investigation.
Finance:
Tasks: ConvFinQA
Performance: The model demonstrated superior comprehension and analysis capabilities, crucial for making informed financial decisions.
Example Application: Providing financial analysts with accurate summaries of market reports and predicting financial trends based on historical data.
Law:
Tasks: CaseHOLD
Performance: The model performed exceptionally well in understanding legal language and concepts, essential for tasks such as legal research and case analysis.
Example Application: Assisting lawyers in summarizing legal documents, identifying relevant case law, and predicting case outcomes.
Domain-Specific Examples:
1. Healthcare:
Usage: Summarizing patient data, predicting treatment outcomes, and assisting in diagnostic processes.
Impact: Reducing the time healthcare professionals spend on administrative tasks, allowing them to focus more on patient care.
2. Finance:
Usage: Analyzing market trends, predicting stock movements, and assessing financial risks.
Impact: Providing financial institutions with deeper insights, leading to better investment decisions and risk management.
3. Legal:
Usage: Researching legal precedents, summarizing case laws, and drafting legal documents.
Impact: Enhancing the efficiency of legal practices by automating repetitive tasks, allowing lawyers to focus on complex legal reasoning.
Continuous Improvement and Future Directions
At Oxford AI Solutions, we believe that the journey of innovation is ongoing. Our approach to reading comprehension-based training is just the beginning. We are committed to further refining this method and exploring new ways to harness the full potential of LLMs. Our goal is to develop AI systems that not only excel in specialized tasks but also adapt and perform across a broad spectrum of applications.
Future Research Directions:
1.Advanced Task Creation:
Developing more sophisticated tasks that challenge the model's understanding and reasoning capabilities.
2. Interdisciplinary Applications:
Expanding the application of our methods to interdisciplinary fields, creating AI systems that can bridge gaps between different domains.
3. Ethical Considerations:
Ensuring that our AI systems are developed with ethical considerations in mind, addressing concerns such as bias, privacy, and transparency.
4. User-Centric Design:
Focusing on the end-users of our AI systems, ensuring that they are user-friendly and tailored to meet the specific needs of different industries.
Join Us in Pioneering AI Innovation
We invite you to join us on this exciting journey of AI innovation. At Oxford AI Solutions, we are dedicated to transforming the way industries operate by integrating advanced AI technologies. By leveraging our expertise in reading comprehension-based training, we aim to set new benchmarks in AI performance and versatility.
Collaborative Opportunities:
Research Partnerships: Collaborate with us on groundbreaking AI research projects.
Industry Applications: Partner with us to develop AI solutions tailored to your specific industry needs.
Educational Initiatives: Engage with us in educational programs to foster the next generation of AI researchers and practitioners.
For more detailed insights into our research and access to our models, code, and data, visit our GitHub repository.
By embracing the power of reading comprehension in training LLMs, Oxford AI Solutions is not just keeping up with the advancements in AI – we are defining them. Join us as we shape the future of artificial intelligence and transform the way industries operate with smarter, more efficient AI solutions.
References
Cheng, D., Huang, S., & Wei, F. (2023). Adapting Large Language Models via Reading Comprehension. ICLR 2024. arXiv