Unleashing the Potential of AI for Spatially Sensitive Nature-Related Financial Risks: Insights from Oxford AI Solutions
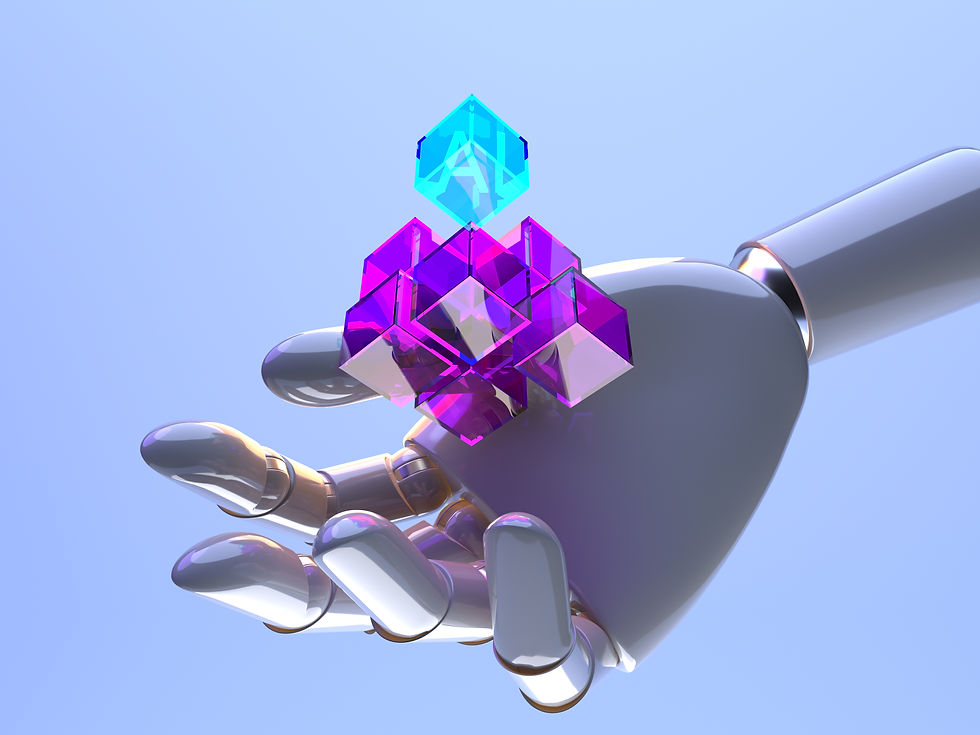
6/6/24, 9:00 PM
In the rapidly evolving landscape of artificial intelligence (AI), Oxford AI Solutions is leading the charge in harnessing the power of AI to address one of the most pressing issues of our time: nature-related financial risks. The recent report, "Assessing the Potential of AI for Spatially Sensitive Nature-Related Financial Risks," highlights our innovative approach and provides a comprehensive analysis of how AI can be leveraged to integrate biodiversity considerations into financial decision-making. This article delves into the key insights from the report, explores practical applications, and envisions future directions for AI in sustainable finance.
Understanding Nature-Related Financial Risks
Nature-related financial risks refer to the potential financial losses that can arise from environmental changes and biodiversity loss. These risks can manifest in various forms, including regulatory changes, reputational damage, and direct impacts on operations. As financial institutions increasingly recognize the importance of sustainable investing, understanding and mitigating these risks has become crucial.
Traditional financial risk assessment methods often fall short in capturing the complexity and spatial sensitivity of nature-related risks. This is where AI comes into play. By leveraging machine learning and advanced data analytics, AI can analyze vast amounts of data, identify patterns, and provide more accurate and granular risk assessments.
The Challenge: Integrating Domain Knowledge with AI
One of the main challenges in assessing nature-related financial risks is the integration of diverse data sources. These sources range from satellite imagery and geospatial data to corporate disclosures and environmental reports. The data is often heterogeneous and varies in quality and granularity, making it difficult to create a comprehensive and reliable risk assessment model.
Moreover, understanding the intricate relationships between different actors in the supply chain and the environment requires sophisticated modeling techniques. Traditional methods may struggle to account for the uncertainties and complexities inherent in these relationships. AI, with its ability to handle large datasets and perform complex analyses, offers a promising solution.
Our Solution: AI-Driven Risk Assessment Models
At Oxford AI Solutions, we have developed a novel approach to tackling these challenges. Our methodology involves transforming raw data into structured, actionable insights through the use of AI-driven models. These models leverage techniques such as probabilistic modeling, Bayesian networks, and machine learning algorithms to provide a comprehensive assessment of nature-related financial risks.
Key Innovations in Our Approach:
1. Probabilistic Modeling:
We use Bayesian networks to model the relationships between different variables and quantify the uncertainties in these relationships. This allows us to simulate various scenarios and assess the potential impacts on biodiversity and financial performance.
For example, in the case of the Brazilian cattle ranching sector, we use a Bayesian model to analyze the environmental impact of ranching activities, regulatory compliance, and the associated reputational risks. This model integrates data from sources such as the Cadastro Ambiental Rural (CAR) and satellite imagery to provide a detailed risk assessment.
2. Geospatial Data Integration:
Incorporating geospatial data enables us to perform asset-level analysis and provide more localized risk assessments. This is crucial for understanding the spatial variability of environmental risks and their impact on financial assets.
In the UK water utility sector, we use geospatial data to assess the risks associated with storm overflow damage and water quality. By analyzing time series data, we can align financial and environmental goals, balancing operational costs with payments for ecosystem services (PES).
3. Machine Learning Algorithms:
Machine learning algorithms help us identify patterns and relationships in large datasets that may not be apparent through traditional analysis methods. These algorithms can continuously learn and adapt, improving their accuracy and predictive capabilities over time.
In our research, we employ machine learning to enhance the centralization and decision-readiness of nature-related data. This involves processing and analyzing diverse datasets to generate actionable insights for financial institutions.
Practical Applications and Case Studies
To illustrate the practical applications of our approach, let's delve into two key case studies highlighted in the report: the Brazilian cattle ranching sector and the UK water utility sector.
Case Study 1: Brazilian Cattle Ranching Sector
The Brazilian cattle ranching sector presents significant nature-related financial risks, particularly due to its impact on deforestation and biodiversity in the Amazon. Our AI-driven model addresses these risks by integrating various data sources and providing a comprehensive risk assessment.
Data Sources:
Cadastro Ambiental Rural (CAR)
Satellite imagery
Environmental impact reports
Regulatory compliance data
Risk Assessment:
The Bayesian model evaluates the environmental impact of ranching activities, including deforestation and habitat destruction.
It assesses regulatory compliance risks, considering potential fines and sanctions for non-compliance with environmental regulations.
The model also analyzes reputational risks, estimating the potential damage to the company’s reputation due to negative environmental impacts.
Impact:
Financial institutions can use these insights to make informed investment decisions, avoiding high-risk ventures and promoting sustainable practices.
Companies can implement more sustainable ranching practices, reducing their environmental footprint and enhancing their market reputation.
Case Study 2: UK Water Utility Sector
The UK water utility sector faces challenges related to water quality and ecosystem health. Our AI-driven model helps address these challenges by providing a detailed risk assessment that balances financial and environmental considerations.
Data Sources:
Geospatial data on water quality and storm overflow events
Time series data on operational expenses and ecosystem service payments
Regulatory compliance data
Risk Assessment:
The model assesses the risks associated with storm overflow damage, analyzing the frequency and severity of overflow events and their impact on water quality.
It evaluates the costs of maintaining water quality standards, balancing these costs with payments for ecosystem services (PES).
Time series analysis enables strategic adjustments in investment decisions, ensuring alignment between financial and environmental goals.
Impact:
Water utility companies can optimize their operations, reducing costs while maintaining high water quality standards.
Financial institutions can invest in companies that demonstrate strong environmental performance, aligning their portfolios with sustainable finance principles.
Future Directions and Recommendations
The report highlights several key areas for future research and development to further enhance the integration of AI in assessing nature-related financial risks.
1. Improving Data Quality and Standardization:
Developing more granular andstandardized data sources is crucial for enhancing the accuracy of AI models.
Collaborative efforts between financial institutions, data providers, and environmental organizations are needed to achieve this goal.
2. Expanding AI Applications:
AI has the potential to be applied to a wider range of nature-related financial risks beyond the case studies presented.
Future research should explore the applicability of AI to other sectors and geographies, further demonstrating its versatility and effectiveness.
3. Enhancing Model Validation and Testing:
The proposed models need to be rigorously tested and validated in real-world scenarios.
Engaging with financial institutions and stakeholders can provide valuable feedback and help refine these models for practical use.
4. Promoting Interdisciplinary Collaboration:
Addressing nature-related financial risks requires expertise from multiple disciplines, including finance, ecology, and AI.
Building interdisciplinary teams and fostering collaboration can enhance the development and implementation of AI solutions.
Conclusion
The integration of AI in assessing nature-related financial risks offers a promising path forward for sustainable finance. By leveraging advanced AI methodologies and robust data sources, financial institutions can better understand and mitigate their impacts on biodiversity and ecosystem services. The innovative approaches developed by Oxford AI Solutions provide a solid foundation for future research and application, paving the way for more sustainable and responsible financial practices.
For more detailed insights into our research and access to our models, code, and data, visit our GitHub repository.
By embracing the power of AI in training LLMs and assessing nature-related financial risks, Oxford AI Solutions is not just keeping up with the advancements in AI – we are defining them. Join us as we shape the future of artificial intelligence and transform the way industries operate with smarter, more efficient AI solutions.